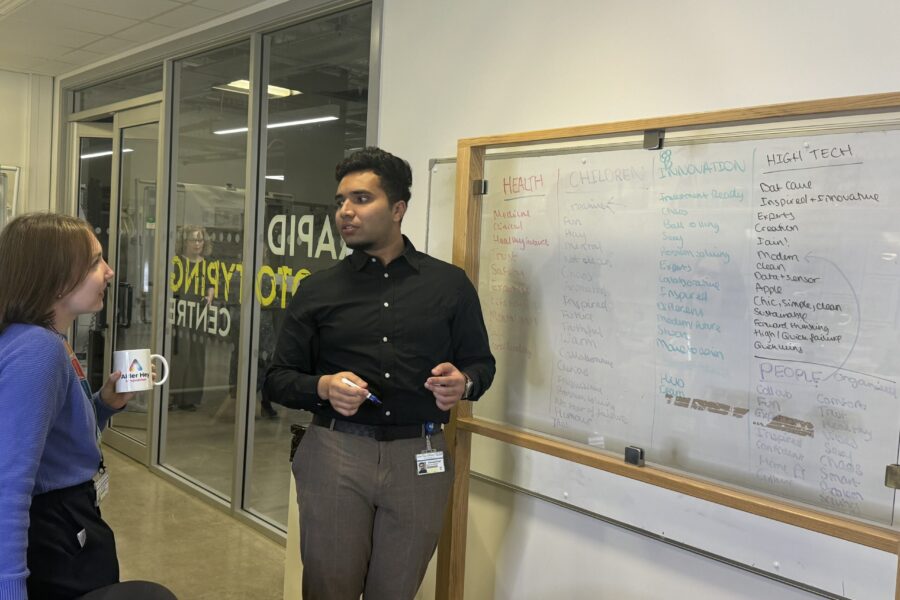
Predicting Patient Surges: Transforming Emergency Care with Data Science
The data science team harnesses AI to analyse vast datasets, including patient records, weather patterns, and public health trends, to develop predictive models that forecast hospital resource needs. By leveraging machine learning, they enable hospitals to plan proactively, improving patient care and operational efficiency through data-driven decision-making.
From Crisis to Precision: Anticipating ED Demand
One winter evening, the Emergency Department (ED) staff at Alder Hey hospital faced an overwhelming influx of patients due to a sudden flu outbreak. Clinicians worked tirelessly, but resources were stretched thin, leading to extended wait times and patient frustration. “We simply weren’t prepared for this volume,” a senior nurse recalled. Today, thanks to an innovative predictive tool, such crises are anticipated and managed with precision, transforming the way ED operates during periods of high demand.
The Challenge: Managing Unpredictable Patient Volumes
Unpredictable patient surges have long challenged EDs, particularly during seasonal illnesses, public events, or emergencies. These sudden spikes often leave staff overworked, resources poorly allocated, and patient care compromised. Without a reliable way to forecast demand, EDs risk inefficiencies that impact patient experience and staff well-being.
A Machine Learning Solution to Predict Patient Demand
To solve this, Alder Hey developed an advanced machine learning model capable of forecasting daily ED attendance. By analysing a combination of historical data, weather trends, and public health statistics, the model identifies patterns and delivers accurate predictions to hospital teams.
The project involved:
- Comprehensive data collection to feed relevant inputs into the model.
- Model training with advanced algorithms to forecast patient volumes accurately.
- Seamless integration into hospital workflows, supporting proactive resource planning and decision-making.
This solution empowers clinicians and managers to allocate resources effectively, ensuring that EDs are prepared for incoming patient surges.
Measurable Outcomes: Accuracy and Efficiency
The predictive model delivered an 85% accuracy rate, enabling Alder Hey to anticipate demand and optimise resource allocation. For example, during a recent flu surge, the model:
- Predicted a 20% rise in patient visits two weeks in advance.
- Allowed the hospital to adjust staffing levels and prepare additional beds.
The results were transformative:
- 30% reduction in patient wait times.
- 20% increase in staff satisfaction due to better workload management.
- 25% improvement in patient satisfaction scores.
This proactive approach has significantly improved both operational efficiency and the overall patient experience.
Current Developments: Expanding Impact Across Healthcare
The Alder Hey data science team continues to enhance the predictive tool with real-time inputs such as public health alerts, ensuring even greater accuracy and responsiveness. Future plans involve sharing the technology across healthcare networks, setting a new standard for resource planning and emergency care preparedness.
By leveraging data science and machine learning, Alder Hey is leading the way in transforming how hospitals predict and respond to patient surges, ensuring efficient, effective, and compassionate care for all.